Big Data Analytics in the Banking Industry
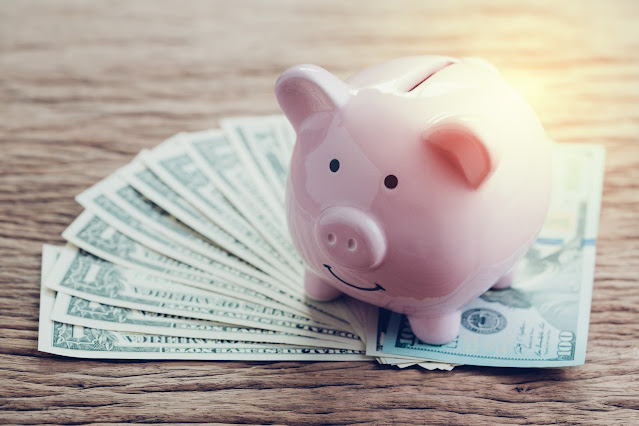
The implementation of big data analytics (BDA) in organizations is a costly and complex undertaking, and given there is no recipe that guarantees a successful implementation and execution, a careful and honest assessment of different aspects of the business must be conducted, from the technology, data collection, the human resources involved in the process, and managerial support (Hajiheydari et al., 2021). Hajiheydari et al. state that approximately 60% to 85% of BDA projects fail not because the technology is bad, but because organizations do not have a clear understanding of how to utilize data analytics to construct achievable business goals, or how to successfully integrate its practice with day-to-day business operations (Sun et al., 2020). BDA offers financial institutions countless benefits, and provides valuable insights to personalize customer service via the analysis of transactional records and other data, including social media information (Flynn, 2020; Sun et al., 2020